Levels of Autonomy and Artificial Intelligence in UAS
Feature Photo: Where to Land Concept. Reprinted from "NASA Autonomous Systems," by Idicula, 2017, NASA. Copyright 2017 by NASA
Researchers from different backgrounds have worked to create a method for measuring the Levels of Autonomy (LoA) in Unmanned Aircraft Systems (UAS) (Elliot & Stewart, 2011). Levels of Autonomy can be measured by breaking the definitions down into levels to show the level in which human operators are required to assist or intervene with the system (Elliot & Stewart, 2011). The range for these levels ranges from 1 to 9 and 10 and above (Elliot & Stewart, 2011).
Levels of Autonomy
Levels of Autonomy from 1 to 3 are considered Low LoA. These systems have very little internal Situation Awareness (SA), and human interaction is the main component for communication and control (Elliot & Stewart, 2011). Low LoA for UAS would have the pilot flying the aircraft with assistance in some areas such as holding a heading, airspeed, or altitude, while the pilot continuously monitors and controls the aircraft as required.
Levels of Autonomy from 4 to 6 are considered Mid LoA. Mid-level LoAs interact with the human operator, approximately 50% of the time (Elliot & Stewart, 2011). The human operators provide the UAS with goals or mission plans, and the UAS must try to execute these commands after the human operator has given the final approval to do so (Elliot & Stewart, 2011). The Mid-level LoAs are different from the low levels in that the operators can create a plan beforehand to have something for the UAS to execute during a flight or mission.
Levels of Autonomy from 7 to 9 are considered High LoA. High-level LoAs have very little interaction from the human operator and do not require human approval to execute its goals (Elliot & Stewart, 2011). The UAS will perform actions and preplanned flight routes without the consent of the operator and has a great understanding of its goal with the capability to conduct high-level, complex decision making (Elliot & Stewart, 2011).
Artificial intelligence and Automation
Artificial Intelligence (AI) is the use of computer-based algorithms that make a determination based on the data that is given to the algorithms (Géron, 2019). These systems are capable of comparing and predicting data to try and predict patterns in the data. This type of AI is typically fed data and determines the most probable outcomes from the data (Géron, 2019). Artificial Intelligence is used to aggregate vast amounts of data and look for patterns that would be biased or difficult if humans were to try and do it themselves (Géron, 2019). An example of AI in UAS is the XQ-58A Valkyrie. The XQ-58A Valkyrie uses artificial intelligence that a manned fighter pilot can command with pushes of a button to send the aircraft forward to uses its sensors while relaying information back to the manned aircraft (Hollings, 2020).
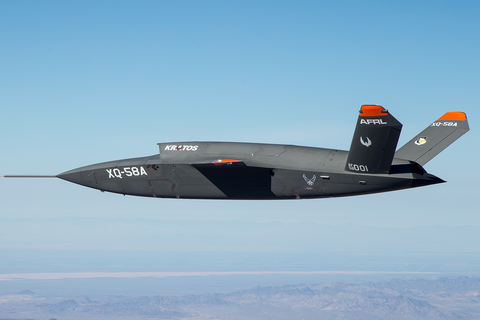
Automation is the completion of repetitive, instructive tasks with preset rules or goals for a system to accomplish (IBM, n.d.). The goals for a system can range from simple to complex depending on the importance of the task (IBM, n.d.). Automation is normally used to complete mundane tasks to allow humans to focus on something other than the task completed. An example of automation for UAS is for the landing gear to lower when the aircraft is beginning its landing process. Lowering the landing gear at a certain time or point frees the pilot for remembering to lower it and allows them to focus on other systems or the approach itself.
NASA Autonomous Systems
The NASA project titled “Determining Optimal Landing Locations in Emergency Situations" researches the capability of an autonomous system to search for and land an aircraft in an emergency. This type of system would be compatible with General aviation, Commercial cargo and passenger vehicles, and UAS (Idicula, 2017). The Where to Land (WTL) system is designed to develop an automated navigation system that can build a decision-making algorithm that would mimic an expert pilot’s decision making by leveraging pre-computer trajectories (Idicula, 2017).
The WTL system uses trajectories using fault locations, maps, terrain, nearby weather, vehicle occupancy, and vehicle capabilities (Idicula, 2017). The intent behind the system is to minimize or remove loss of life, property, and assets in the event of an emergency (Idicula, 2017). The WTL system also takes into account the damage or problems with the aircraft to determine viable landing sights to accommodate the aircraft (Idicula, Akametalu, Chen, Tomlin, Ding, & Hook, 2015).
Where to Land and UAS
According to Meuleau, Neukom, Plaunt, Smith, D. E., & Smith, T. B (2011), a WTL system would expand the capabilities of UAS in the National Airspace System (NAS). Where to Land capabilities for UAS can consist of a method for lost link scenarios and flight termination scenarios. In the event of a UAS that is flown using a high LoA, the aircraft could experience a corrupted mission plan onboard the aircraft. A WTL system would enable the aircraft to land safely at an airfield without having to lose the aircraft due to a corrupt mission file.
Another situation where WTL would support UAS is a loss link scenario. In the event of a loss link scenario, the UAS could determine the safest course of action and fly the aircraft to the airfield and land with a lower probability of damaging equipment or causing casualties from a crash. With an increase in capabilities for UAS with a WTL system, it would give lawmakers and advocates some talking points to show the public that the systems can be safely operated and landed in the event of an emergency.
Future of Artificial Intelligence for UAS
In an article written by Sean McPherson (2020) at C4ISRNet, Sean explains that the future of the Department of Defense(DoD) requirements for the use of AI. The DoD has defined ethical principles that AI must follow for it to be accepted and utilized in future systems.
The ethical principles listed by the DoD are:
Responsible. DoD personnel will exercise appropriate levels of judgment and care while remaining responsible for the development, deployment, and use of AI capabilities.
Equitable. The Department will take deliberate steps to minimize unintended bias in AI capabilities.
Traceable. The Department’s AI capabilities will be developed and deployed such that relevant personnel possess an appropriate understanding of the technology, development processes, and operational methods applicable to AI capabilities, including with transparent and auditable methodologies, data sources, and design procedures and documentation.
Reliable. The Department’s AI capabilities will have explicit, well-defined uses, and the safety, security, and effectiveness of such capabilities will be subject to testing and assurance within those defined uses across their entire life-cycles.
Governable. The Department will design and engineer AI capabilities to fulfill their intended functions while possessing the ability to detect and avoid unintended consequences, and the ability to disengage or deactivate deployed systems that demonstrate unintended behavior.
DoD. (2020, February 25). DOD Adopts Ethical Principles for Artificial Intelligence. Retrieved May 04, 2020, from https://www.defense.gov/Newsroom/Releases/Release/Article/2091996/dod-adopts-ethical-principles-for-artificial-
intelligence/
The ethical principles by the DoD have created a framework for future innovators and creators of machine learning AI. As Sean McPherson (2020) explains, the principles are high level, which allows for each agency inside the DoD to determine the full definition and explanation for each principle along with how they will approach it. Some Unmanned Aircraft System designs already utilize AI to help perform portions of its duties. The XQ-58 will utilize these types of technology to ensure the 'loyal wingman' is performing its duties appropriately based on the mission requirements that day.
References
DoD. (2020, February 25). DOD Adopts Ethical Principles for Artificial Intelligence. Retrieved May 04, 2020, from https://www.defense.gov/Newsroom/Releases/Release/Article/2091996/dod-adopts-ethical-principles-for-artificial-intelligence/
Elliott, L. J., & Stewart, B. (2011). Automation and Autonomy in Unmanned Aircraft Systems (pp. 100-117). In R. K. Barnhart, S. B. Hottman, D. M. Marshall, & E. Shappee (Eds.), Introduction to Unmanned Aircraft Systems. Available from https://ebookcentral-proquest-com.ezproxy.libproxy.db.erau.edu/lib/erau/reader.action?docID=1449438&ppg=1
Géron, A. (2019). Hands-On Machine Learning with Scikit-Learn, Keras, and TensorFlow: Concepts, Tools, and Techniques to Build Intelligent Systems. O'Reilly Media.
Hollings, A. (2020, March 17). This Experimental Drone Could Change America's War Strategy. Retrieved from https://www.popularmechanics.com/military/a31122720/kratos-xq58a-valkyrie-future/
IBM. (n.d.). What is automation? Retrieved from https://www.ibm.com/topics/automation
Idicula, J. (2017, April 28). Autonomous System. Retrieved from https://www.nasa.gov/feature/autonomous-systems#landing
Idicula, J., Akametalu, K., Chen, M., Tomlin, C., Ding, J., & Hook, L. (2015). Where to Land: A Reachability Based Forced Landing Algorithm for Aircraft Engine Out Scenarios.
McPherson, S. (2020, May 03). Ensuring the Pentagon follows ethics for artificial intelligence. Retrieved from https://www.c4isrnet.com/opinion/2020/05/03/ensuring-the-pentagon-follows-ethics-for-artificial-intelligence/?utm_source=Sailthru
Meuleau, N. F., Neukom, C., Plaunt, C. J., Smith, D. E., & Smith, T. B. (2011). The emergency landing planner experiment.